GetJenny Blog Posts
Chatbot Case Studies for Media and Entertainment Companies
How can media and entertainment companies keep up with customers? Learn how chatbots help service teams stay on track and serve customers better.
Read the article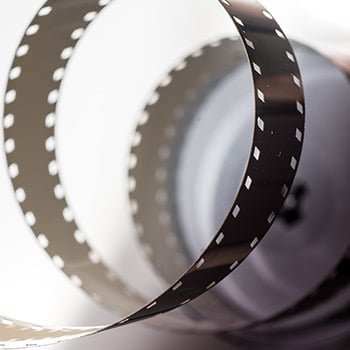
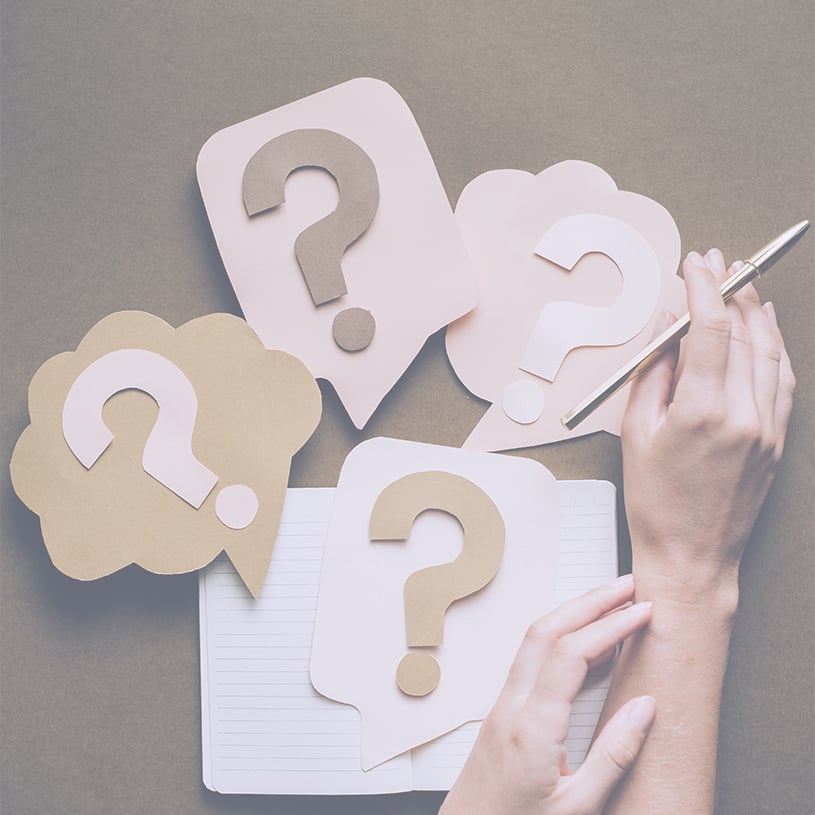
4 Things You Need to Know When Considering Chatbots
Ersin walks through the surprising opportunities potential chatbot customers miss in their chatbot project planning.
Read moreInfographic: Customer Experience in the Energy Sector
Key insights for customer experience in the Energy and Utilities sector in a handy one-sheet. Get the facts and numbers in one place.
Read more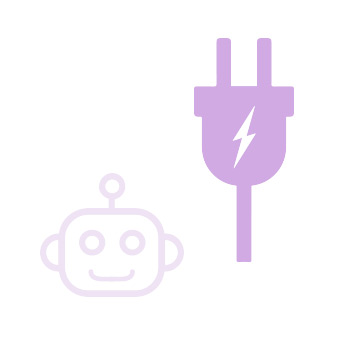
16 Real-Life Examples of Energy Companies Utilizing Industry Trends
How European Energy, Gas and Utility companies are utilizing industry trends to grow business in times of uncertainty? Let's take a look at the examples.
Read more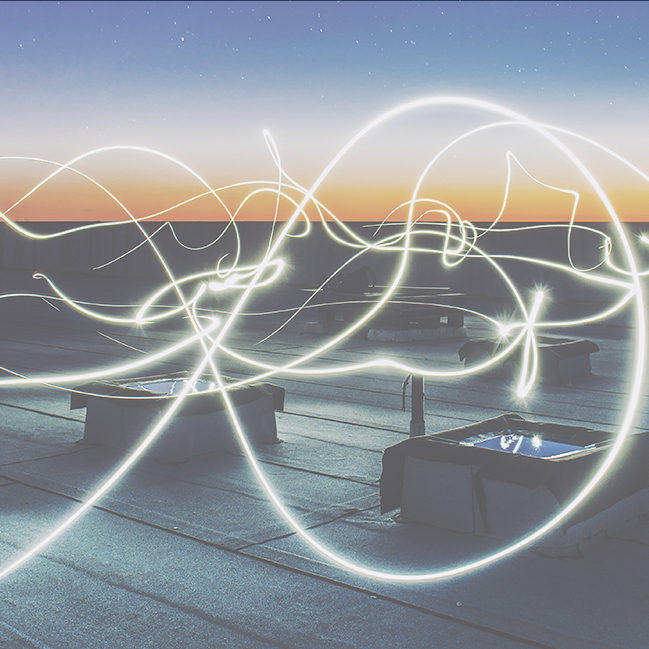
How Chatbots Can Make Your Business Stand Out in a Saturated Market
Having problems standing out in the industry? Then chatbots could help! Here's why you should use chatbots to increase your bottom line.
Read more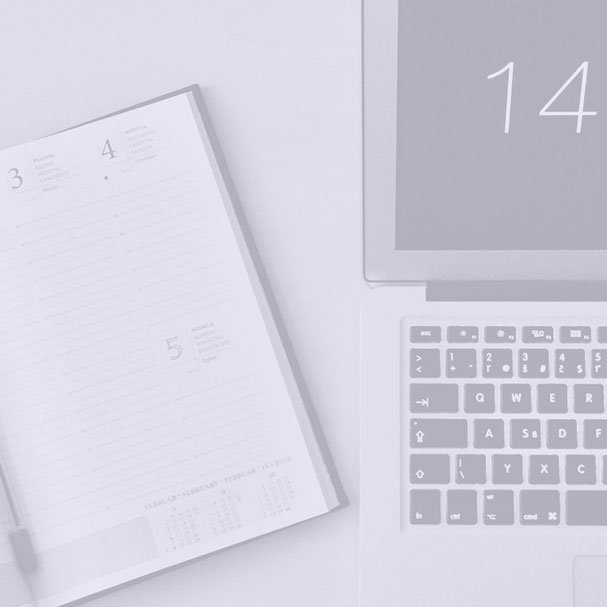
Must-Have AI Chatbot Features for Better Customer Experience
Are you considering an AI chatbot? This guide helps you ask the right questions to chatbot vendors about chatbot features when you compare options.
Read more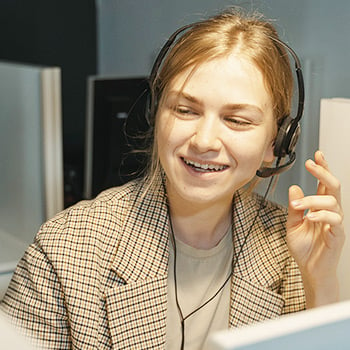
How Chatbot Metrics Influence Customer Service Outcomes
Does your chatbot influence customer satisfaction? How about team capacity or agent satisfaction? Find the chatbot metrics affecting your customer service.
Read more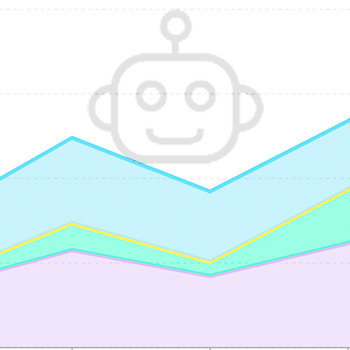
Top Chatbot Case Studies and Their Real-Life Results
Learn how brands use chatbots in real life. This article on chatbot case studies outlines how businesses improve customer satisfaction daily with AI.
Read more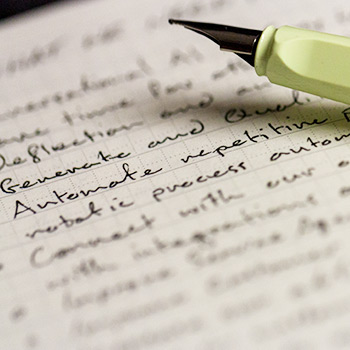
7 Essential Marketing Trends for 2021
This article outlines the biggest marketing trends in 2021 and helps you pick the right trends to fulfil YOUR business goals.
Read more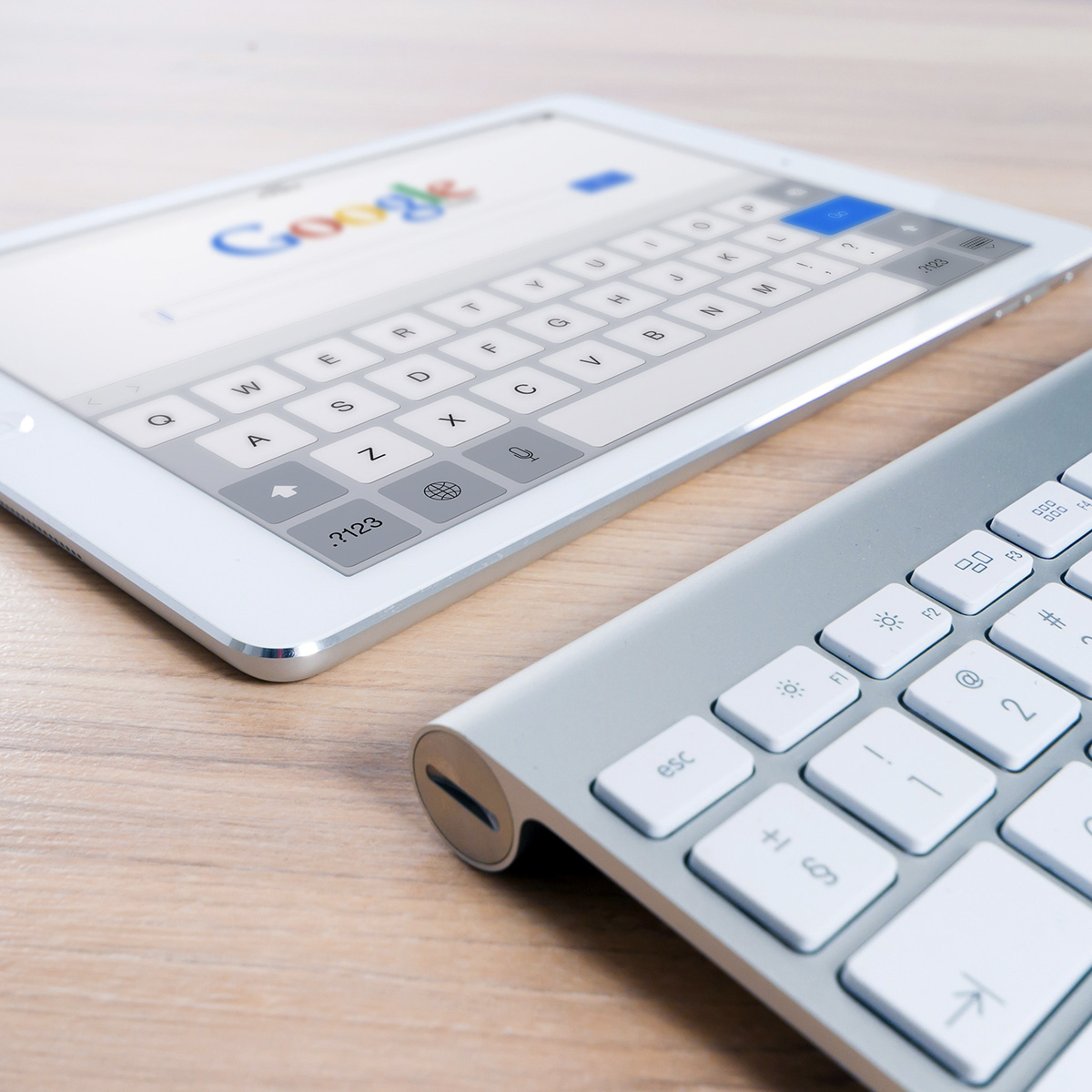
How to Reconnect with Customers Using AI Customer Service Chatbots
Customers want fast, comprehensive solutions. Learn from this in-depth article on how to reconnect customers using AI customer service chatbots.
Read more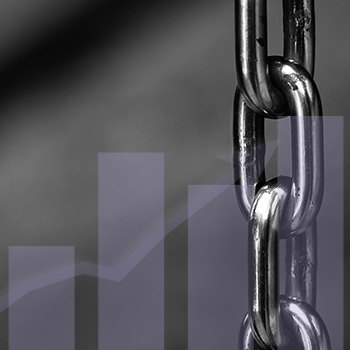
Balancing Automated and Hands-On Tools for Customer Experience
As demand for digital services grows, need for automated solutions is clear, but it's essential to learn where to balance automation with human support.
Read more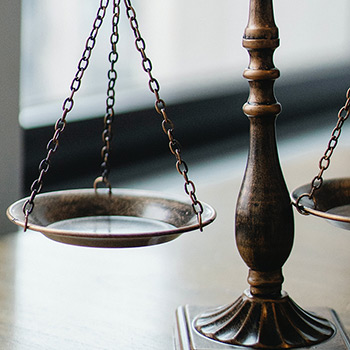
Can Pop-Ups be Good for Customer Experience?
Are pop-ups good or bad? It depends. Learn a few ways to make pop-ups better for customer experience and to minimise use, while still achieving your CTRs.
Read more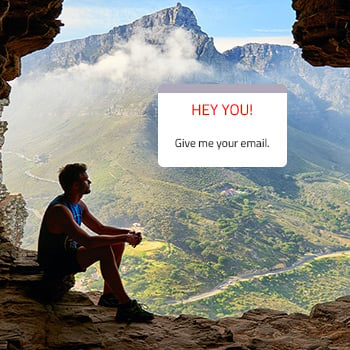
Why AI Needs Human Supervision: My Journey to GetJenny
Kimmo Valtonen's thoughts on the concerns of unsupervised AI and how his journey has brought him to GetJenny.
Read more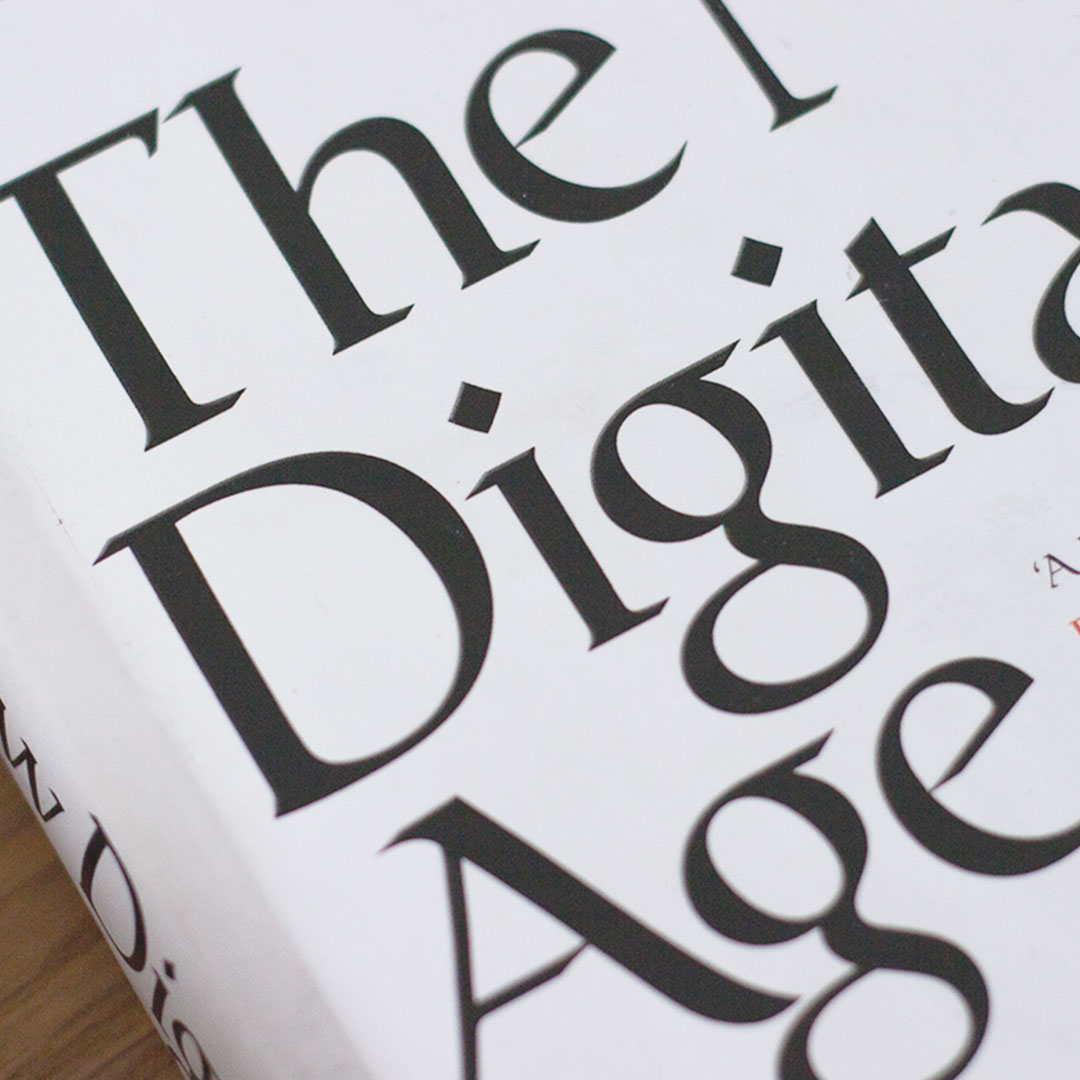
3.5 Ways to Make Great First Impressions Online
You have 0.05 seconds to make it or break it. Get the 3.5 CX tips to make a great first impression online and win over visitors on your website.
Read more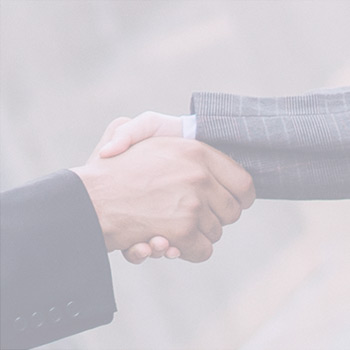
7 Growth Hacks to Use Chatbots for Customer Service
Retain customers and sustainably grow your brand with these 7 effective tips for using customer service chatbots.
Read more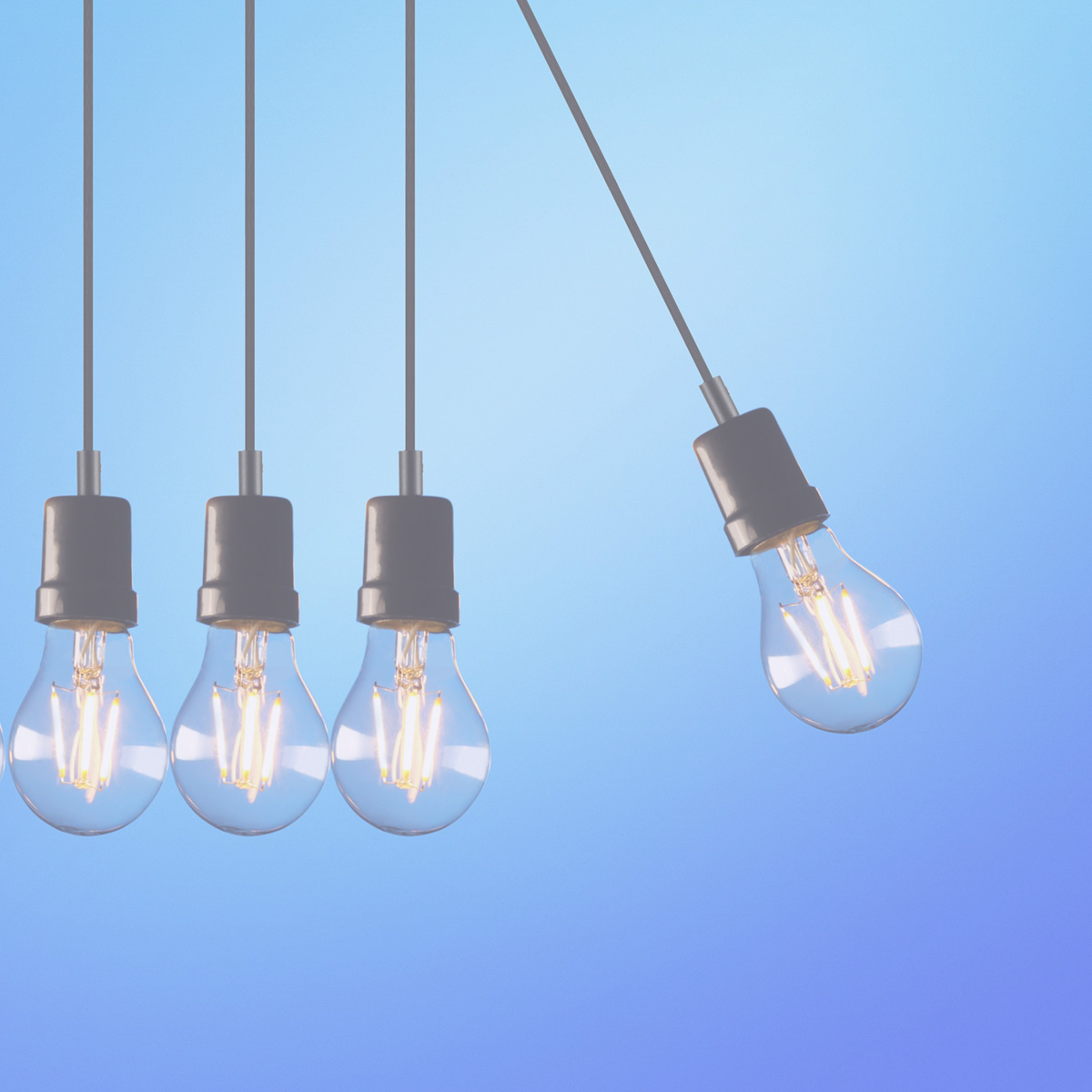
More blog posts
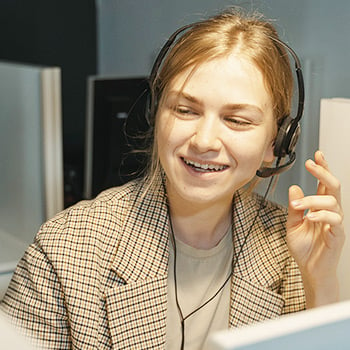
Must-Have AI Chatbot Features for Better Customer Experience
So you've decided to improve your customer service with AI chatbots?
Read more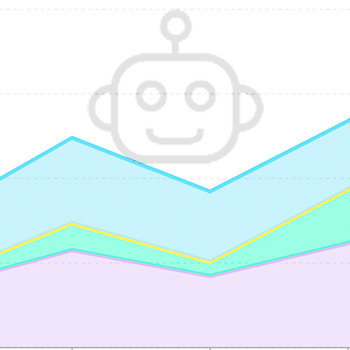
How Chatbot Metrics Influence Customer Service Outcomes
If you get a chatbot on your website, how will it affect your overall...
Read more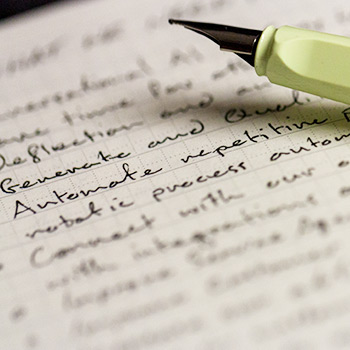
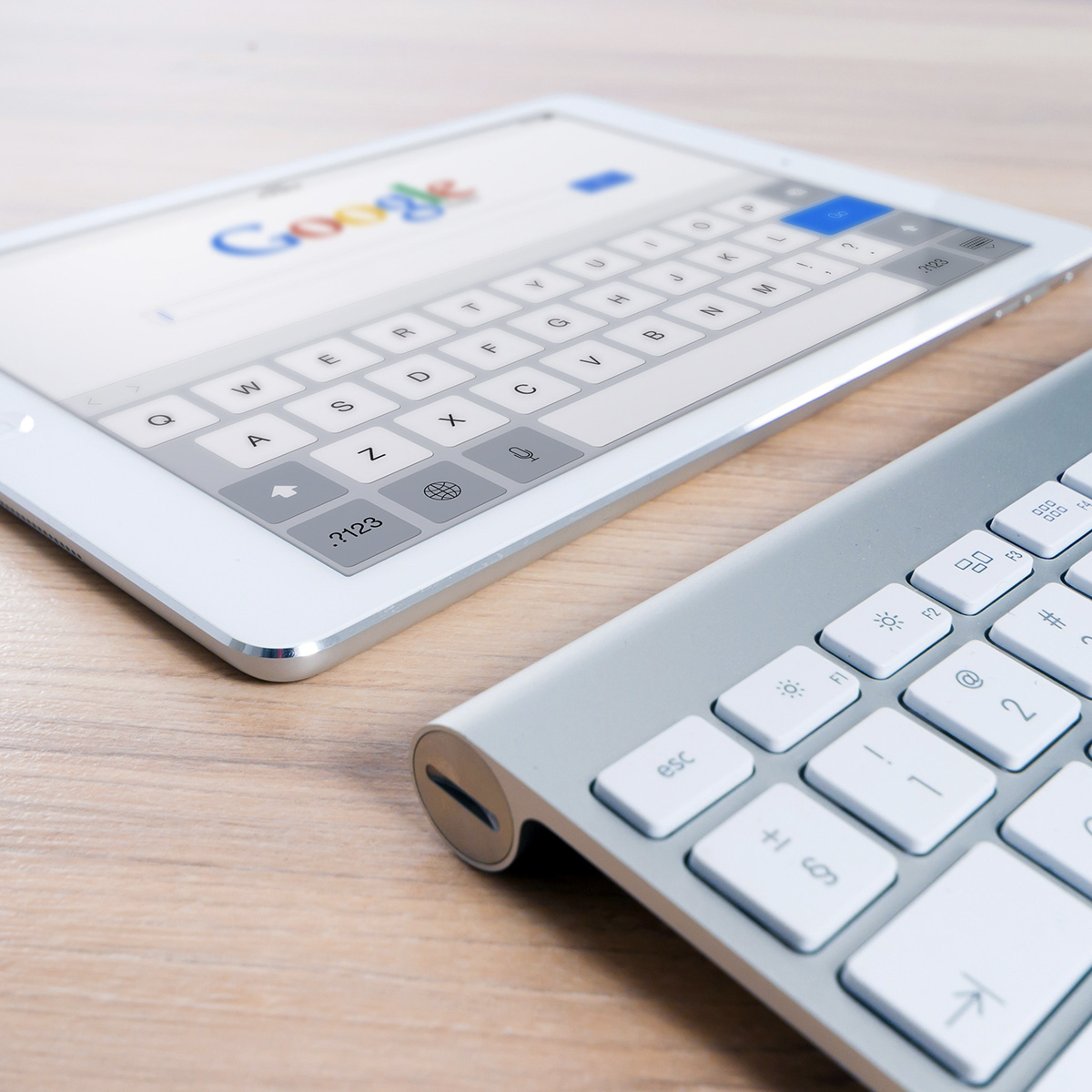
7 Essential Marketing Trends for 2021
For many businesses 2020 was not the best year to test out new...
Read more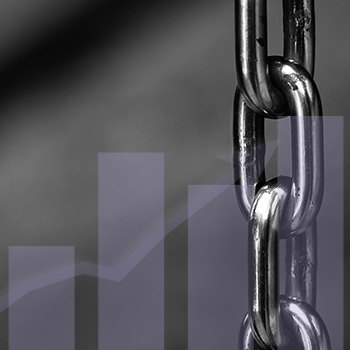
How to Reconnect with Customers Using AI Customer Service Chatbots
To have a successful business, you need customers. And for your...
Read more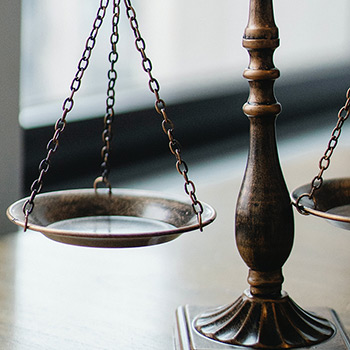
Balancing Automated and Hands-On Tools for Customer Experience
Let's get one thing sorted right off the bat: you need both online....
Read more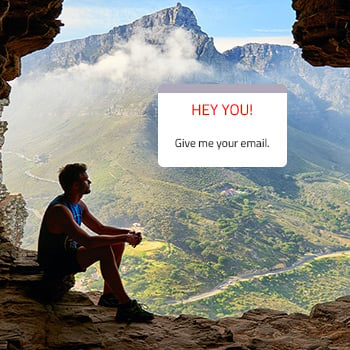
Can Pop-Ups be Good for Customer Experience?
There's a lot of hate for pop-ups.
A quick google search on whether...
Read more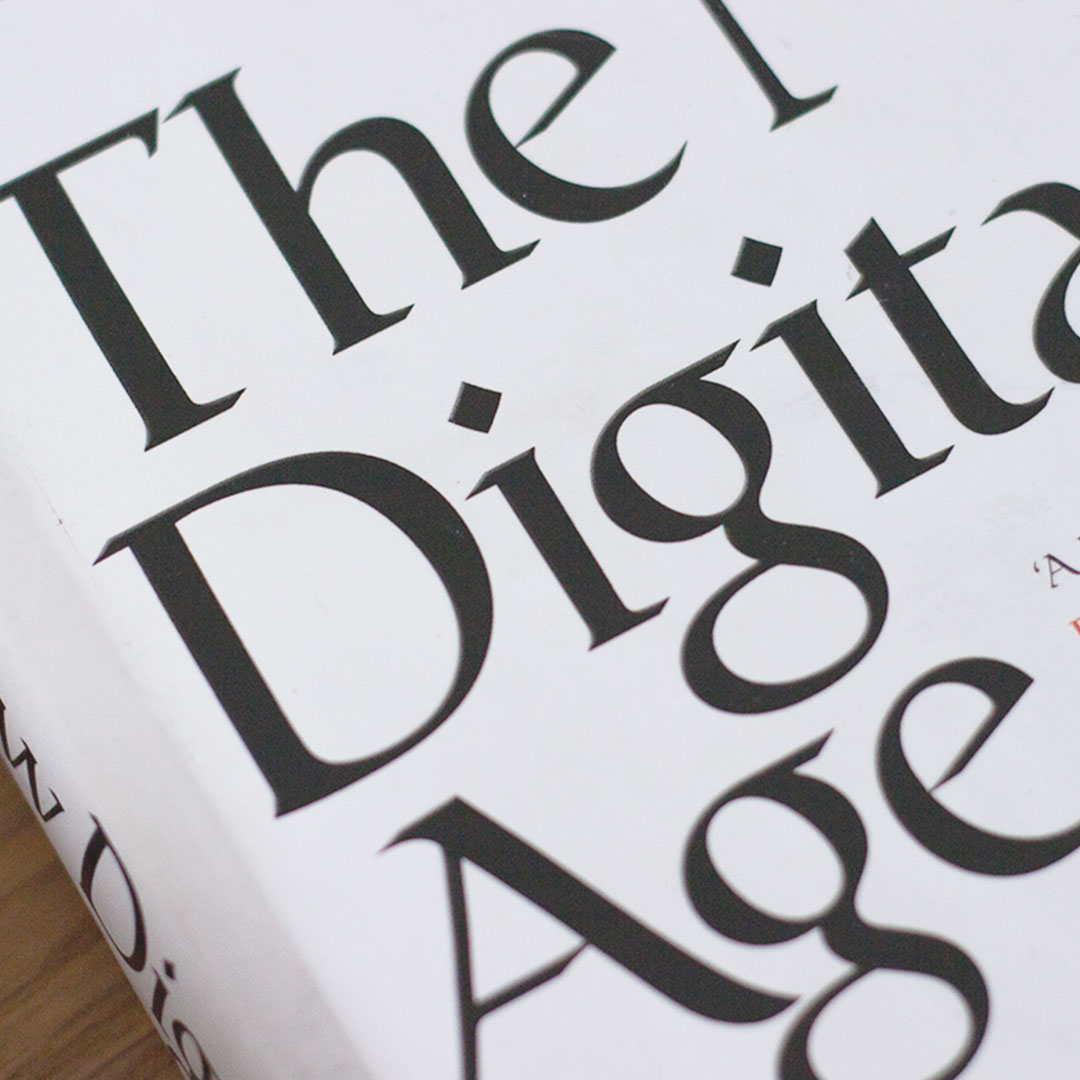
Why AI Needs Human Supervision: My Journey to GetJenny
Joining a new company is always exciting. Doubly so when you join a...
Read more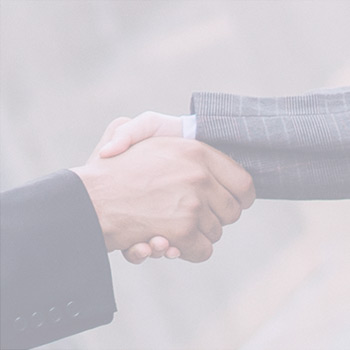
3.5 Ways to Make Great First Impressions Online
Tell me what you do. You have seven seconds.
Go.
Read more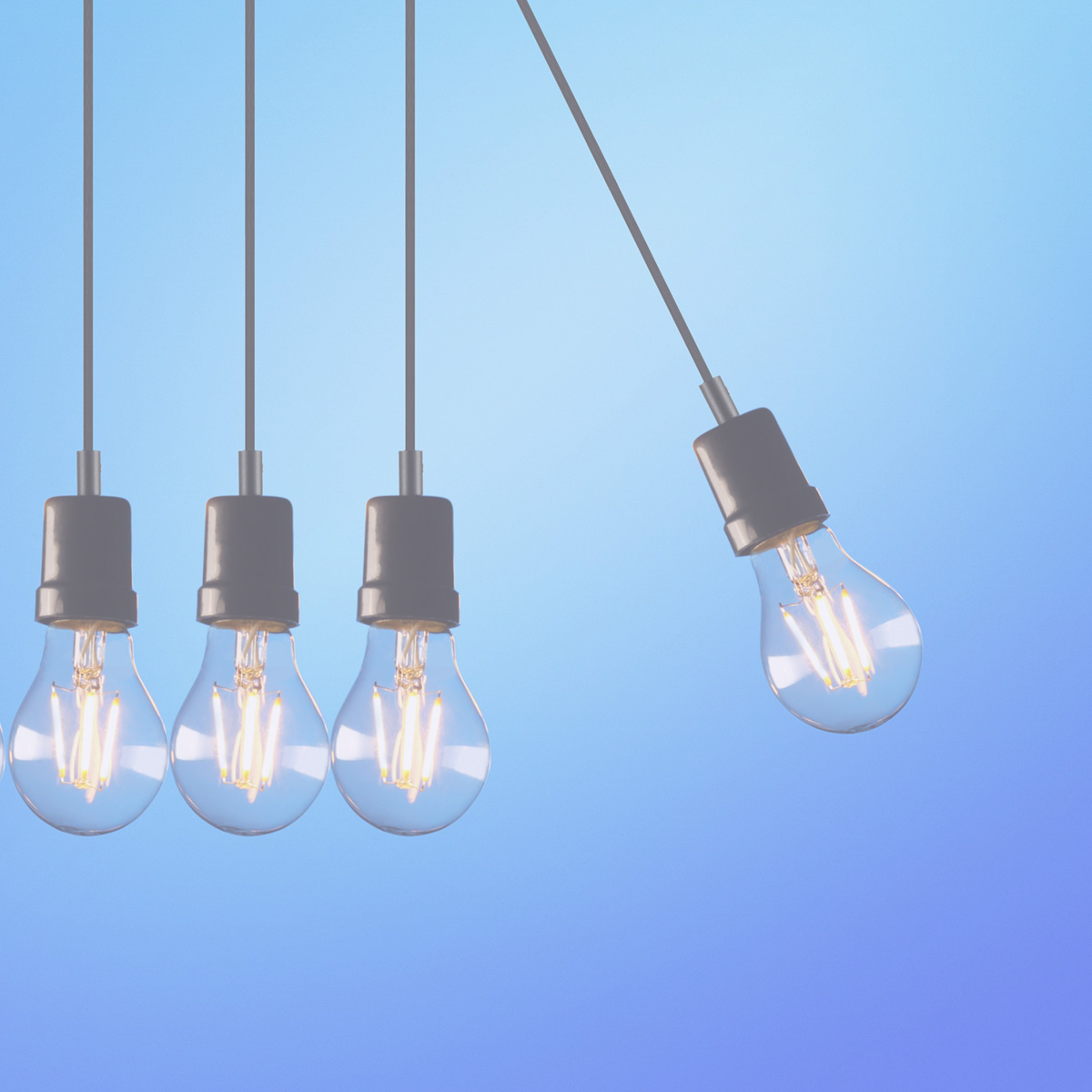
7 Growth Hacks to Use Chatbots for Customer Service
When people talk about growth hacking, many of us think about...
Read more